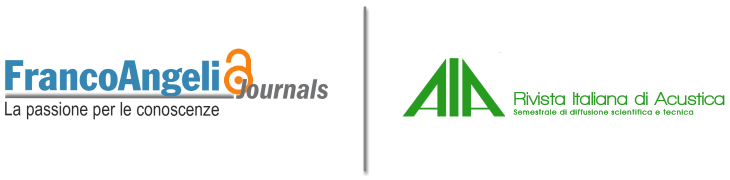
The constant growth of power calculation and data storage focuses the interest on long-term monitoring. Such a large amount of data paves the way for machine learning techniques. Using sophisticated algorithms, usually based on statistics, broadens the analysis capability in complex scenarios. The present work proposes a machine learning-based method to separate, identify, and measure different coexisting sound sources through sound level meters in real-world scenarios. Four case studies show the application of the proposed method. Two case studies concern monitoring university lessons to separate the speech levels of the teacher from the students' chatter. In this way, the focus of students during lessons can be measured. Further, two case studies concern monitoring two offices with multiple workstations to separate noise sources from workers’ speech.